You probably already know that Google is constantly changing its ranking algorithm to offer users the best possible results.
On August 7, 2023, the search engine announced that it was researching a new ranking framework called Term Weighting BERT (TW-BERT), which is designed to improve search results.
In this article, we find out what TW-BERT is and how it could help Google improve its search results.
Google’s announcement of the TW-BERT search framework
Google has developed a research paper that introduces a fascinating framework known as TW-BERT. Its main function is to improve search rankings without incurring substantial modifications.
TW-BERT presents itself as a query term weighting context that combines two paradigms to enhance search results.
It harmonizes with existing query expansion models while increasing their efficiency. What’s more, its introduction into a new framework requires only minimal modifications.
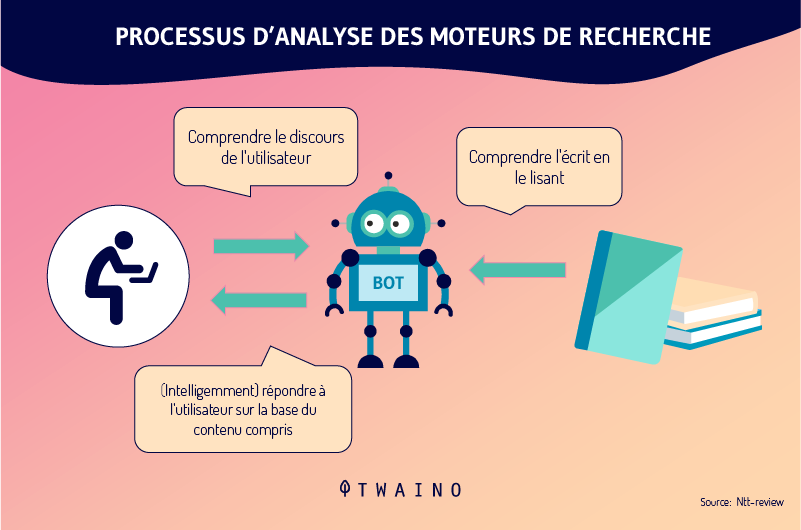
Term Weighting BERT (TW-BERT) is an impressive ranking framework revealed by Google. It optimizes search results and can be easily integrated into existing ranking systems.
Although Google has not confirmed the exploitation of TW-BERT, this new framework represents a major advance that will improve ranking processes in various areas, including query expansion.
Notable contributors to TW-BERT include Marc Najork, a leading researcher at Google DeepMind and former Senior Director of Search Engineering at Google Research.
TW-BERT – What’s it all about?
TW-BERT is a ranking context that assigns scores, also known as weights, to the words contained in a search query. The aim is to determine more precisely which specific pages are relevant to that particular query.
When it comes to query expansion, TW-BERT is very useful. This expansion is a process that reformulates a search query or adds words to it(for example, adding the word “home” to the search “bodybuilding exercise“) with the aim of more adequately matching the search query with the documents.
The research paper talks about two different search methods: one based on statistics and the other oriented towards deep learning models.
According to the researchers,
“These statistics-based retrieval methods enable an efficient search that adapts to corpus size and generalizes to new domains. However, terms are weighted independently and do not take into account the context of the query as a whole.
For this problem, deep learning models can perform this contextualization on the query to provide better representations for individual terms.“
To illustrate the weighting of search terms via TW-BERT, the researchers take the example of the query “Nike running shoes”. Each term in this query is given a score, or “weighting”, which helps to understand the query as it was issued by the user.
In this illustration, the word “Nike” is considered important. Naturally, this word will receive a higher score. The researchers conclude by stressing the importance of ensuring that the word “Nike” receives sufficient weighting while displaying running shoes in the final results.
The other challenge is to understand the connection between the words “running” and “shoes”. This implies that the weighting should be increased when the two words are combined to form the phrase “running shoes”, rather than weighting each word separately.
TW-BERT: The solution to the limitations of current frameworks
The research paper discusses the inherent limitations of current weighting when it comes to query variability, and points out that statistically-based weighting methods underperform in “shoot from scratch” situations.
Learning from scratch refers to a model’s ability to solve a problem for which it has not been trained.
The researchers also presented a summary of the inherent limitations of current term expansion methods.
Term expansion refers to the use of synonyms to find more answers to search queries, or when another word is inferred.
For example, when someone searches for “chicken soup”, this implies “chicken soup recipe”.
The researchers describe the shortcomings of current approaches in the following terms:
“… these auxiliary scoring functions do not take into account the additional weighting steps implemented by the scoring functions used in existing extractors, such as query statistics, document statistics, and hyperparameter values.
This can alter the original distribution of weights assigned to terms during final evaluation and retrieval.”
Subsequently, the researchers argue that deep learning has its own challenges in the form of deployment complexity and unpredictable behavior when it encounters new domains for which it has not been pre-trained.
This is where TW-BERT comes in.
Why TW-BERT?
The proposed solution resembles a hybrid approach.
The researchers write:
“To bridge this gap, we leverage the robustness of existing lexical extractors with the contextual representations of text provided by deep models.
Lexical extractors already assign weights to query n-gram terms during search.
We exploit a linguistic model at this stage of the pipeline to assign appropriate weights to query n-gram terms.
This method of weighting BERT terms (TW-BERT) is globally optimized using the same scoring functions used in the pipeline to ensure consistency between training and retrieval.
This improves search when using the term weights produced by a TW-BERT model, while maintaining an information retrieval infrastructure similar to its existing production counterpart.”
The TW-BERT algorithm assigns weights to queries to provide a more accurate relevance score on which the rest of the ranking process can then work.
What the standard lexical search system looks like
This diagram illustrates the flow of data in a standard lexical search system.
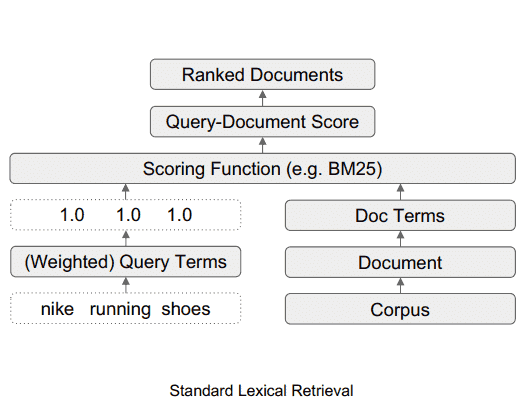
Source: searchenginejournal
What the TW-BERT search system looks like
This diagram shows how the TW-BERT tool fits into a search framework.
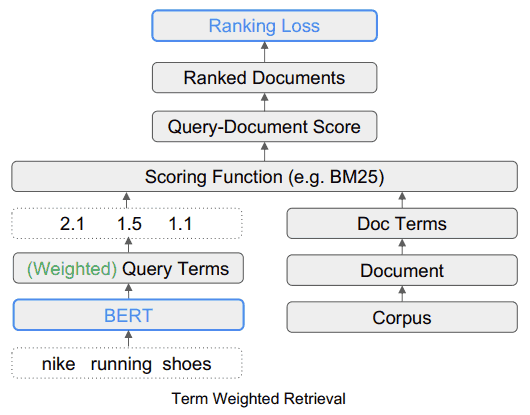
Source: searchenginejournal
TW-BERT’s ease of deployment
One of the advantages of TW-BERT is that it can be easily introduced into the current information search classification process, as a plug-and-play component.
“This allows us to deploy our term weightings directly into an information retrieval system during search.
This differs from previous weighting methods that require additional tuning of an extractor’s parameters to achieve optimal extraction performance, as they optimize term weights obtained by heuristics instead of end-to-end optimization.”
A key factor in TW-BERT’s ease of deployment is that it does not require the use of specialized software or hardware upgrades. This capability makes integrating TW-BERT into a rankingalgorithm system simple.
Has Google already added TW-BERT to its ranking algorithm?
In terms of its applicability to Google’s ranking algorithm, TW-BERT’s ease of integration suggests that Google may have adopted the mechanism into its algorithm structure.
The framework could be integrated into the algorithm’s ranking system without requiring a complete update of the core algorithm.
On the other hand, certain algorithms that are inefficient or offer no improvement, although intriguing in their design, might not be retained for Google’s ranking algorithm. On the other hand, particularly effective algorithms, such as TW-BERT, are attracting attention.
TW-BERT has proven highly effective in enhancing the capabilities of current ranking systems, making it a viable candidate for adaptation by Google.
If Google has already implemented TW-BERT, this could explain the ranking fluctuations reported by SEO monitoring tools and the search marketing community over the past month.
While Google only makes public a limited number of ranking changes, those that have a significant impact, there is as yet no official confirmation that Google has integrated TW-BERT.
We can only speculate on its likelihood, based on the notable improvements to the accuracy of the information retrieval system and the practicality of its use.
To sum up
TW-BERT is a major SEO innovation that could change the way Google analyzes and ranks web pages. TW-BERT has not yet been officially integrated into Google’s algorithm, but may be in the near future.